To predict the \( y \)-value for \( x \)-value 69 you run a regression analysis on a bivariate set of data, after checking the data points in the scatter plot had few outliers and were roughly grouped around a (straight) line. Use significance level of \( \alpha=.05 \) to assess the strength of the linear correlation. Linear Regression results are \[ \hat{y}=-0.2 x+4.1 \] You also find \( \bar{x}=10.7 \) and \( \bar{y}=2 \). To predict the \( y \)-value when \( x=69 \), \( y \approx \) \( \bar{x}=10.7 \) \( \hat{y}=-0.2 \cdot 69+4.1=-9.7 \) \( \bar{y}=2 \) All of these None of these
Solución de tutoría real
Responder
Solución
Revisado y aprobado por el equipo de tutoría de UpStudy


Beyond the Answer
When you plug in \( x = 69 \) into the regression equation \( \hat{y} = -0.2 \cdot 69 + 4.1 \), you get \( \hat{y} = -9.7 \). This prediction might raise some eyebrows since this value falls significantly below the mean of \( \bar{y} = 2 \). Essentially, while regression analysis provides valuable insights, it's crucial to remember that predictions based on extrapolated data might lead to unrealistic or non-meaningful outcomes. It’s essential to consider the context of your data when interpreting these outcomes. If your dataset primarily consisted of \( x \)-values around 10.7, predicting beyond the range (like at 69) can yield numbers that don’t necessarily reflect the real-world scenario. Always keep in mind that extrapolation tends to amplify error, so tread carefully when making predictions well outside the observed range of your dataset!
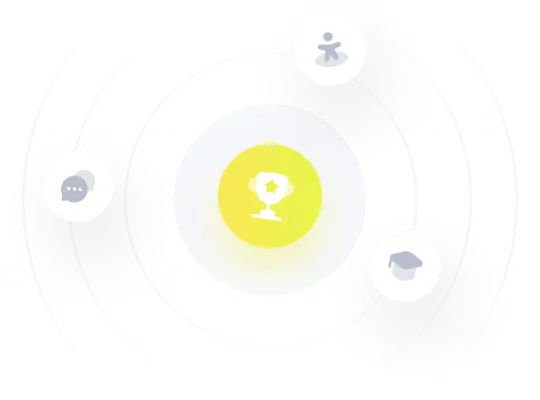